Machine learning and gene regulation
High throughput experiments such as next generation sequencing are often used to answer simple biological questions; “which genes are more expressed in breast cancer compared to normal?”.
Given the huge amount of information generated for each experiment, this is equivalent to having a privileged access to an oracle and asking “what time is it?”.
Machine learning is an excellent tool for discovering hidden information in large amounts of data. These not only allow life scientists to get better answers but also to generate novel hypotheses.
Our lab looks for opportunities in medical and fundamental biology data where information theory and machine learning can make a substantial impact.
A few examples of our discoveries include using Shannon’s Entropy to discover transcriptional disorder in cancer (PLoS CB, 2008), simulating a biologists behavior to identify a method to detect microRNA targets (Nature Methods, 2009) and using novel bioinformatics strategies to discover the impact of introns on gene expression (Cell, 2013; Genome Biology 2017; Nature Communications 2017).
Symposium Artificial Intelligence in Biology and Health, October 2018: Back to the symposium with all the videos of the speakers
We are holding a one-day symposium that will bring together world-class experts from the fields of Artificial Intelligence, Biology and health. The speakers have been selected based on their expertise in their field and their ability to speak to a broad audience. Our objective is to enable the audience to move outside of their own comfort zone and discover recent breakthroughs in a different field of science. The theme of the day and subject of our roundtable discussion will be on how to get the fields of AI, biology, and health to work together in science and academia. This experiment is free but requires registration as places are limited.
Videos of the Symposium
Publications of the team
TALC: Transcription Aware Long Read Correction
Lucile Broseus, Aubin Thomas, Andrew J Oldfield, Dany Severac, Emeric Dubois, William Ritchie
GECKO is a genetic algorithm to classify and explore high throughput sequencing data
Aubin Thomas, Sylvain Barriere, Lucile Broseus, Julie Brooke, Claudio Lorenzi, Jean-Philippe Villemin, Gregory Beurier, Robert Sabatier, Christelle Reynes, Alban Mancheron, William Ritchie
Exploring the Roles of CREBRF and TRIM2 in the Regulation of Angiogenesis by High-Density Lipoproteins.
Wong NKP, Cheung H, Solly EL, Vanags LZ, Ritchie W, Nicholls SJ, Ng MKC, Bursill CA, Tan JTM
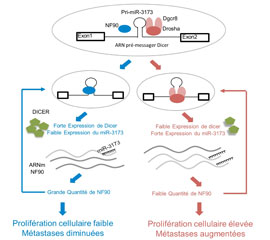
An NF90/NF110-mediated feedback amplification loop regulates dicer expression and controls ovarian carcinoma progression.
Barbier J, Chen X, Sanchez G, Cai M, Helsmoortel M, Higuchi T, Giraud P, Contreras X, Yuan G, Feng Z, Nait-Saidi R, Deas O, Bluy L, Judde JG, Rouquier S, Ritchie W, Sakamoto S, Xie D, Kiernan R
Intron retention is regulated by altered MeCP2-mediated splicing factor recruitment
Wong JJ, Gao D, Nguyen TV, Kwok CT, van Geldermalsen M, Middleton R, Pinello N, Thoeng A, Nagarajah R, Holst J, Ritchie W, Rasko JEJ
IRFinder: assessing the impact of intron retention on mammalian gene expression
Middleton R, Gao D, Thomas A, Singh B, Au A, Wong JJ, Bomane A, Cosson B, Eyras E, Rasko JE, Ritchie W.
microRNA Target Prediction
Ritchie W
Intron retention enhances gene regulatory complexity in vertebrates.
Schmitz U, Pinello N, Jia F, Alasmari S, Ritchie W, Keightley MC, Shini S, Lieschke GJ, Wong JJ, Rasko JEJ
Thèses et hdr
Design and implementation of bioinformatic tools for RNA sequencing data analysis 20/10/2021
Defended by Claudio Lorenzi on 20/10/2021
Methods for studying intron retention from second and third generation sequencing data 13/11/2020
Defended by Lucile Broseus on 13-11-2020 under the supervision of William Ritchie - Montpellier